Vortrag: Rethinking Probabilities: Why Corpus Frequencies Cannot Capture Speakers' Dynamic Linguistic Behaviour
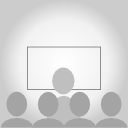
Researchers often refer to information in terms of the information content of a word, as outlined in information theory by Shannon (1948) and Hartley (1928). It is standard practice to calculate the information content of a word by using the occurrence probability taken from language corpora. This approach aggregates word frequencies across contexts, creating the illusion that words occur uniformly. However, this observation does not align with empirical findings which show bursty behaviour in language, where words with initially low occurrence probabilities often exhibit a higher likelihood of reoccurrence once introduced in a specific context (Katz, 1996). The potential impact of burstiness has been largely overlooked in probabilistic research on language processing.
In our elicitation study participants were instructed to describe object movements to an absent listener. We investigated whether speakers demonstrate sensitivity to dynamic probabilities and adjust their nominal reference phrases accordingly.
Based on the findings of Krauss and Weinheimer (1964), we predicted that speakers adjust the length of their reference phrases based on lexical frequency, resulting in longer reference phrases for relatively lower frequency nouns. However, our data could not sustain this hypothesis which we attribute to the absence of a discourse partner in our study. Consistent with proposals that prenominal adjectives increase noun predictability (Dye et al., 2018), we correctly predicted that participants would show a higher probability for noun modification at first mention than at subsequent mentions of the referent. These findings indicate that speakers re-evaluate a word’s probability to reoccur in context, supporting the idea that speakers manage uncertainty and informativity not only by using the overall statistical distribution of words but also by dynamically adjusting expectations of occurrence probabilities even in the absence of a discourse partner.
Our findings indicate that considering burstiness and dynamic probabilities is essential for advancing research on language processing.
Info
Tag:
10.05.2024
Anfangszeit:
14:45
Dauer:
00:30
Raum:
Squid (33.0.008)
Track:
Neuro- and Psycholinguistics
Sprache:
en
Links:
Dateien
- Kemper_Rethinking Probabilities_Abstract StuTS 2024.pdf
- Kemper_Rethinking Probabilities_Abstract StuTS 2024.docx
Feedback
Uns interessiert Ihre Meinung! Wie fanden Sie diese Veranstaltung?
Gleichzeitige Events
ReferentInnen
![]() |
Santina S. Kemper |