Lecture: Extracting Scenario Knowledge in LLMs: A Case Study with ChatGPT in English and Spanish
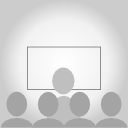
Since last year, the launch of ChatGPT (OpenAI, 2023) has revolutionised the state of the art in Large Language Models (LLMs). One can only imagine the possibilities that such a powerful tool can bring, since LLMs may illustrate how humans understand words through the notion of scenario, or "knowledge about the world that can involve groups of events and entities that often appear together" (Erk and Herbelot, 2022, p. 22). This is the basis of how humans build mental representations of words, since every word is linked to one or multiple scenarios. Understanding how LLMs extract scenario knowledge information may thus provide insight into how humans understand language. The purpose of this study was to find the best manner of extracting scenario information from words from LLMs in a human-readable form. To achieve this, we tested the generated texts of 15 words with ChatGPT in English and Spanish. Our objective was to try to generate a meaningful description that contained all the required elements per scenario and, if available, overlapping frames. Scenario descriptions were used as criteria for a successful generation, through a combination of WordNet (Princeton University, 2010) definitions and FrameNet (University of California, Berkeley, 2006), frame descriptions. Generated texts sufficed if they resembled the description, and contained all core elements and at least one non-core element. Overall, ChatGPT demonstrated the ability to generate scenario descriptions with all core elements of the word's main frame in 73.33% of English trials and 64.44% of Spanish trials. Furthermore, all of these descriptions also contained at least one non-core element. For words linked to multiple frames, ChatGPT consistently generated texts that included multiple frames (100%). We hope that the present study will contribute to the current state of the art in LLMs, especially in exploring how LLMs represent linguistic knowledge and perform knowledge extraction.
References:
Katrin Erk and Aurelie Herbelot. 2022. How to marry a star: probabilistic constraints for meaning in context. ArXiv:2009.07936.
OpenAI. 2023. ChatGPT (May 24 Version).
Princeton University. 2010. About WordNet.
University of California, Berkeley. 2006. The Berkeley FrameNet Project.
Info
Day:
2023-10-27
Start time:
11:30
Duration:
00:25
Room:
NIG Raum 2
Track:
Computational Linguistics
Language:
en
Links:
Files
Feedback
Click here to let us know how you liked this event.
Concurrent Events
Speakers
![]() |
Cristina Reguera-Gómez |
![]() |
Mila Brandsen |