Talk: The Language of Fairness How NLP Can Reflect and Reinforce Organizational Bias
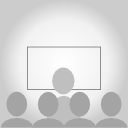
As Natural Language Processing (NLP) tools become more pervasive in recruitment, claims of fairness and objectivity often mask the subtle ways language can insidiously encode and reproduce organizational bias. This paper draws on empirical insights from an interdisciplinary study of AI-driven hiring systems to examine how NLP algorithms can mirror biased linguistic patterns—embedded in resumes, job postings, and training data. Despite being framed as neutral, such systems often reflect historical hiring inequalities, leading to unintended but impactful outcome biases that disproportionately affect underrepresented candidates (Hardy et al., 2022, p. 659). This research uses Socio-Technical Systems (STS) theory as a guiding framework for examining how both the technical design of NLP models and the social context of their use contribute to fairness challenges. Transformer-based models (e.g., BERT) are analyzed not only for their semantic matching capabilities but also for their vulnerability to inherited linguistic bias. As Blodgett et al. (2020) argue, language technologies often reproduce power asymmetries because they are built on biased corpora and lack contextual sensitivity (p. 5456). Using empirical examples from organizational settings, the study shows how NLP technologies—if designed and audited inconsiderately—can entrench institutional norms and power relationships even when they seem to reduce human subjectivity. Spanning computational linguistics and ethical recruitment practice, the article contributes to ongoing debates about AI fairness with some practical insights on how recruitment technologies can be both efficient and fair.
Info
Day:
2025-05-17
Start time:
11:50
Duration:
00:30
Room:
GWZ 4.216
Track:
Other
Language:
en
Links:
Files
Concurrent Events
Speakers
![]() |
Roya Samad-Zada |