Lecture: The rest is syntax
(Non-)compositionality in semantic phrase embedding
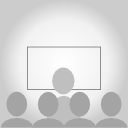
I present preliminary research on compositional phrase embeddings using a novel tree LSTM autoencoder. I will outline the necessary theoretical background for grammar-theoretical, neurolinguistic and NLP notions of (non-)compositionality and introduce the tree LSTM architecture. Finally I will go over my experimental set-up and the work ahead.
In this short talk I present my preliminary work on surprisal measures for text reuse retrieval.
One problem that deliniates (literary) text reuse from plagiarism is the use of wordplay and meaning altering substitutions on the quoted phrase. Arthur Golding, for example, writes "Instead of legs, to both her sides stick fingers long and fine: The rest is belly.” when describing the fate of Arachne in his translation of Ovid’s Metamorphoses (1567), which is later invoked in the dying words of Hamlet (”The rest is silence.”). Shakespeare is referencing Ovid (through Golding) to get to give his tragedy a mythological, heroic vibe. To do this, he is performing a substitution from belly to silence to match his scene.
To put it in concrete terms, there is a transposition from the global intertextual dialogic context the phrase is retrieved from to the local context of the borrowing text. My hypothesis is that the resulting violation of the global context should be surprising.
Surprisal here can be thought of in the information-theoretical sense of information content. If these borrowed phrases are lexically embedded “as is”, we expect with high probability to see the phrase unaltered. Any transformation therefore should have a lower probability. Traditionally these probabilities are calculated by way of word distributions and corpus analysis.
In psycholinguistic research surprisal is often measured through cloze tests. These closely resemble the masked learning methods used by state-of-the-art language models during training. I expect to be able to extract an approximation of surprise by measuring embedding distances between predicted and actual tokens from these models. Because of the inherent attention mechanism in these models I should also be able to trace the predictions to varying windows of context.
The amplitude of the N400 component of the event-related potential (ERP) in response to linguistic stimuli also has a high correlation with the kind of semantic surprisal I am interested in in this work. Recently there have been major advances in modelling the N400 component directly through sentence gestalt models.
I plan to use these different measures of surprisal on an annotated data set on text reuse in Early Modern English and a word mover’s distance based classifier trained on the task.
In this talk I will outline the necessary theoretical background for these three approaches and the overall task. Finally I will present my thoughts on the experimental set-up and share preliminary results.
Info
Day:
2022-05-26
Start time:
14:00
Duration:
00:30
Room:
Living Lab (1.34)
Track:
Computational Linguistics
Language:
en
Links:
Feedback
Click here to let us know how you liked this event.
Concurrent Events
Speakers
![]() |
Victor Zimmermann |