Lecture: Hate Speech Detection on Code-Switched Data
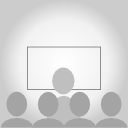
The following presentation will focus on adapting a pre-trained BERT model to a code-switched dataset to be able to create a hate speech detection model handling code-switching.
With half of the human population having a social media account, it has never been easier to express your thoughts and opinions in front of a wider audience. As a consequence, verbal aggressions such as hate speech have increased dramatically and are not only harming the mental health of individuals but also damaging the relationship between different ethnical groups due to offensive and abusive content. Therefore it became important to lawmakers and social media platforms to filter this kind of behaviour online. For instance, each social media platform has its own policies to remove hate speech based on their definition. While Facebook defines hate speech as violent or dehumanising speech or calls for exclusion or segregation of people based on protected characteristics such as race, gender, etc., Twitter sums up everything which promotes violence or threats against other people. In the past, this kind of content has been removed manually, but with 500 million tweets and 4.3 billion Facebook messages everyday, manual hate speech detection has become impractical and ineffective. Consequently, an automated approach for hate speech detection is required. Therefore several machine learning models have been developed for monolingual hate speech detection in the past, with models based on pre-trained language models, such as BERT, being the most successful ones.
Conversations where individuals switch between different languages are becoming more and more frequent in the context of globalisation and migration. This phenomenon is called code-switching. In the past, when working with code-switched data, it was not only difficult to find an appropriate data source but also most models in information extraction are not able to handle more than two languages. As it is common in multilingual societies like India to use code-switching to convey opinions online, models which were specifically trained on code-switched data are required to be able to prevent hate speech online.
For this reason, the following thesis will focus on hate speech detection on code-switched data by adapting a BERT based hate speech detection model to code-switched data. Due to its prominence in urban Hindi-speaking nations, the language combination of Hindi and English will be used as a dataset.
Info
Day:
2022-05-27
Start time:
12:00
Duration:
00:30
Room:
Schuurman (1.22)
Track:
Computational Linguistics
Language:
en
Links:
Files
Feedback
Click here to let us know how you liked this event.
Concurrent Events
Speakers
![]() |
Barbara Kovačić |