Lecture: Spraakherkenning, wa is da? — Bias in Flemish Speech Recognition
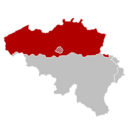
Sociolinguistic factors such as age and gender have been shown to impact the performance of various automatic speech recognition (ASR) models. Previous research has touched upon such performance discrepancies, uncovering biases in ASR models, but has often focused on the English language. However, as these systems are used worldwide, finding biases in different languages is of high importance. With this thesis, I extend recent research by Feng, Kudina, Halpern, and Scharenborg (2021), who sought to find biases based on age, region, gender, and non-nativeness in a Dutch ASR model. Like Feng et al. (2021), I use the Netherlandic Dutch data from the Spoken Dutch Corpus to train a hybrid deep neural network-hidden Markov model (DNN-HMM). However, the previous study did not take into account the various regional variants of Belgian Dutch, which is also known as Flemish, e.g., West Flemish, and Brabantian. I therefore evaluate the model using the Flemish data from the JASMIN-CGN corpus. The evaluation confirms a bias against speakers from West Flanders and Limburg, as well as against children, male speakers, and non-native speakers. In addition, the discussion of the findings includes an analysis of the most misrecognized phonemes. The current study contributes to a better understanding of bias, and subsequently inclusivity, in ASR.
-
Feng, S., Kudina, O., Halpern, B. M., & Scharenborg, O. (2021). Quantifying bias in automatic speech recognition. arXiv preprint arXiv:2103.15122.
At 71. StuTS + 31. TaCoS I presented my research proposal. At 72. StuTS I will present my finished work.
Info
Day:
2022-11-05
Start time:
14:15
Duration:
00:30
Room:
Wiwi-Bunker —Room 4047
Track:
Computational Linguistics
Language:
en
Links:
Feedback
Click here to let us know how you liked this event.
Concurrent Events
Speakers
![]() |
Aaricia Herygers |